The MePheSTO vision is to break the scientific ground for the next generation of precision Psychiatry through social interaction analysis based on artificial intelligence methods.
Within the MePheSTO project, we develop clinically valid phenotypes for psychiatric disorders based on multimodal inputs from clinical social interactions. Focus lies on schizophrenia and depression. Starting point is a multi-centre, multinational thus multi-language study [König et al, 2022] that collects data from social interactions between clinicians and patients. The multilingual corpus consists of video-, audio recordings along with biometric data recorded with Kinect cameras and wrist-worn bio-sensors. Data is then transcribed and annotated with gestures, body movements, facial expression etc and so that phenotypes can be identified using machine-learning methods.
For context, digital phenotype are derived from digital data streams rather then from biological blood samples. Thereby, multiple scientific domains collaborate: computer vision, speech analysis, behavioural science, medicine etc.
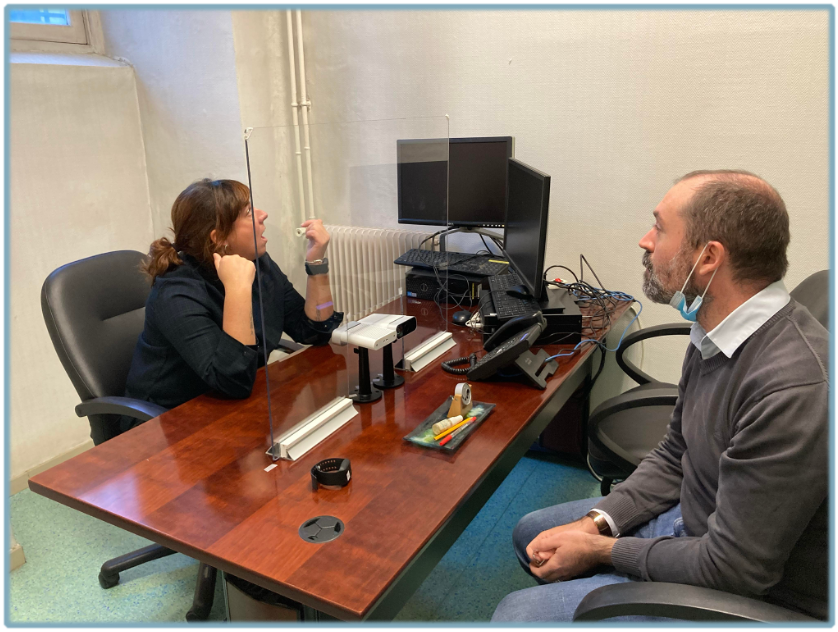
Recording Setup
Short Term Objectives | Establishing an ambitious multidisciplinary research core group between INRIA and DFKI. As well as laying the foundation for generating future value from scientific excellence. Pushing the interdisciplinary research on novel medical AI applications by leveraging INRIA’s (STARS) expertise in computer vision and DFKI’s knowledge on speech and dialogue analysis with the goal of clinical interaction analysis.
- Design a multi-site data collection study
- Establish multimodal digital biomarkers for psychiatric pathologies
- Demonstrate innovative markers in clinical scenarios
Long Term Objectives | Building novel scientific expertise at the interface of computer vision, computational linguistics, psychology, sociology and medicine (neurology & psychiatry). From this scientific ecosystem, generate applied and innovation projects (e.g. via EIT Health, other funding schemes) and economic value (e.g. start-up creation & industrial projects).
- Trusted Research Environments: developing and certifying trusted research environments for maintaining highly sensitive data, see SEMLA (https://semla.dfki.de)
- Establish contract negotiations for future French-German Collaboration
Four Research Cases:
Positive Symptoms in Schizophrenia | Objective measurement of positive symptoms in Schizophrenia through automatic speech analysis
Supporting Differential Diagnosis | Supporting differential Diagnosis for major depressive episode eitology through combined analysis of video, audio and physiology data
Quantify Therapeutic Alliance | Which multimodal measure are indicative for interaction quality levels between clinicians and patients?
Relapse Prediction | Prediction of symptoms progress & treatment response; definition of digital phenotypes (over time)
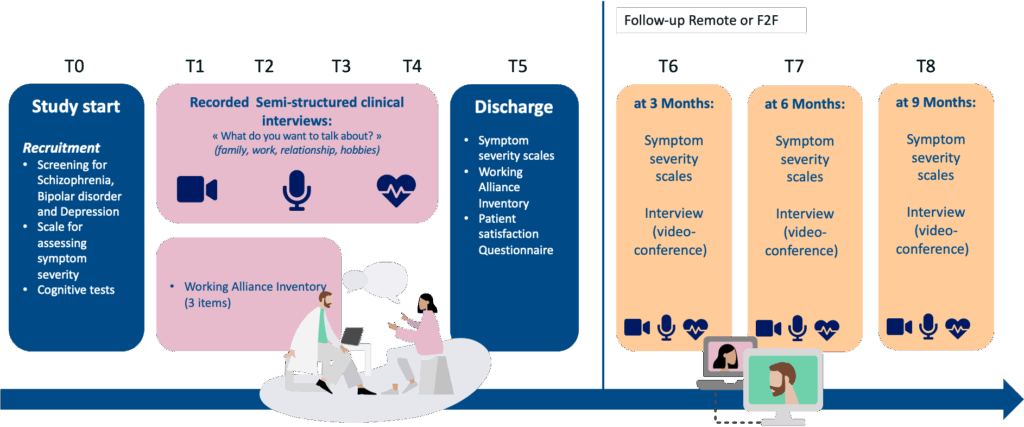
Study Protocol
Partners:
• DFKI (Dr. Jan Alexandersson, Dr. Johannes Tröger, Dr. Philipp Müller, COS)
• INRIA, Nice (Dr. François Bremond, STARS team)
• INRIA, Nancy (Dr. Maxime Amblard, SEMAGRAMME team)
• Nice University Clinic (Prof. Dr. Philippe Robert, University Côte d’Azur)
• University Clinic of Saarland (Prof. Dr. Matthias Riemenschneider)
• Centre Psychothérapique de Nancy (Prof. Dr Vincent Laprevote)
• Centre Hospitalier Montperrin Aix-en-Provence (Sophie Barthelemy, Phd, psychologist)
• Dept. Of Psychiatry, University of Oldenburg, Karl Jaspers Klinik (Prof. Dr. René
Hurlemann)
Publications:
- König, A., Müller, P., Tröger, J., Lindsay, H., Alexandersson, J., Hinze, J., … & Hurlemann, R. (2022). Multimodal phenotyping of psychiatric disorders from social interaction: Protocol of a clinical multicenter prospective study. Personalized Medicine in Psychiatry, 33, 100094.
- Balazia, M., Müller, P., Tánczos, Á. L., Liechtenstein, A. V., & Brémond, F. (2022, October). Bodily Behaviors in Social Interaction: Novel Annotations and State-of-the-Art Evaluation. In Proceedings of the 30th ACM International Conference on Multimedia (pp. 70-79).
- Agrawal, T., Balazia, M., Müller, P., & Brémond, F. (2023). Multimodal Vision Transformers with Forced Attention for Behavior Analysis. In Proceedings of the IEEE/CVF Winter Conference on Applications of Computer Vision (pp. 3392-3402).
- Labbé, C., König, A., Lindsay, H., Linz, N., Tröger, J., & Robert, P. (2022). Dementia vs Depression: new methods for differential diagnosis using automatic speech analysis. Alzheimer’s & Dementia, 18, e064486.
- Lindsay, H., Tröger, J., Mina, M. M., Müller, P., Linz, N., Alexandersson, J., & Ramakers, I. (2022, June). Generating Synthetic Clinical Speech Data Through Simulated ASR Deletion Error. In Proceedings of the RaPID Workshop-Resources and ProcessIng of linguistic, para-linguistic and extra-linguistic Data from people with various forms of cognitive/psychiatric/developmental impairments-within the 13th Language Resources and Evaluation Conference (pp. 9-16).
- Ter Huurne, D. B., Ramakers, I. H., Linz, N., König, A., Langel, K., Lindsay, H., … & de Vugt, M. (2021). Clinical use of deep speech parameters derived from the semantic verbal fluency task. Alzheimer’s & Dementia, 17, e050380.
- Lindsay, H., Müller, P., Kröger, I., Tröger, J., Linz, N., König, A., … & Ramakers, I. H. (2021, September). Multilingual Learning for Mild Cognitive Impairment Screening from a Clinical Speech Task. In Proceedings of the International Conference on Recent Advances in Natural Language Processing (RANLP 2021) (pp. 830-838).
- König, A., Zeghari, R., Guerchouche, R., Tran, M. D., Bremond, F., Linz, N., … & Robert, P. (2021). Remote cognitive assessment of older adults in rural areas by telemedicine and automatic speech and video analysis: protocol for a cross-over feasibility study. BMJ open, 11(9), e047083.
- Lindsay, H., Mueller, P., Linz, N., Zeghari, R., Mina, M. M., König, A., & Tröger, J. (2021, June). Dissociating semantic and phonemic search strategies in the phonemic verbal fluency task in early dementia. In Proceedings of the Seventh Workshop on Computational Linguistics and Clinical Psychology: Improving Access (pp. 32-44).
- König, A., Riviere, K., Linz, N., Lindsay, H., Elbaum, J., Fabre, R., … & Robert, P. (2021). Measuring stress in health professionals over the phone using automatic speech analysis during the COVID-19 pandemic: observational pilot study. Journal of Medical Internet Research, 23(4), e24191.
- Lindsay, H., Tröger, J., & König, A. (2021). Language Impairment in Alzheimer’s Disease—Robust and Explainable Evidence for AD-Related Deterioration of Spontaneous Speech Through Multilingual Machine Learning. Frontiers in aging neuroscience, 228.
- Ettore, E., Müller, P., Hinze, J., Riemenschneider, M., Benoit, M., Giordana, B., … & König, A. (2023). Digital phenotyping for differential diagnosis of major depressive episode: narrative review. JMIR Mental Health, 10, e37225.
- Müller, P., Balazia, M., Baur, T., Dietz, M., Heimerl, A., Schiller, D., … & Bulling, A. (2023). MultiMediate’23: Engagement Estimation and Bodily Behaviour Recognition in Social Interactions. Proc. ACM International Conference on Multimedia.
- Amer, A., Bhuvaneshwara, C., Addluri, G. K., Shaik, M. M., Bonde, V., & Müller, P. (2023). Backchannel Detection and Agreement Estimation from Video with Transformer Networks. Proc. IEEE Joint Conference on Neural Networks.
- Sood, E., Kögel, F., Müller, P., Thomas, D., Bâce, M., & Bulling, A. (2023). Multimodal integration of human-like attention in visual question answering. In Proceedings of the IEEE/CVF Conference on Computer Vision and Pattern Recognition (pp. 2647-2657).
- Sood, E., Shi, L., Bortoletto, M., Wang, Y., Müller, P., & Bulling, A. (2023). Improving Neural Saliency Prediction with a Cognitive Model of Human Visual Attention. Proc. of the 45th Annual Conference of the Cognitive Science Society.
- Müller, P., Dietz, M., Schiller, D., Thomas, D., Zhang, G., Gebhard, P., … & Bulling, A. (2021, October). MultiMediate: Multi-modal Group Behaviour Analysis for Artificial Mediation. In Proceedings of the 29th ACM International Conference on Multimedia (pp. 4878-4882).
- Penzkofer, A., Müller, P., Bühler, F., Mayer, S., & Bulling, A. (2021, October). ConAn: A Usable Tool for Multimodal Conversation Analysis. In Proceedings of the 2021 International Conference on Multimodal Interaction (pp. 341-351).
- Strohm, F., Sood, E., Mayer, S., Müller, P., Bâce, M., & Bulling, A. (2021). Neural Photofit: Gaze-based Mental Image Reconstruction. In Proceedings of the IEEE/CVF International Conference on Computer Vision (pp. 245-254).
- Müller, P., Staal, S., Bâce, M., & Bulling, A. (2022, April). Designing for Noticeability: Understanding the Impact of Visual Importance on Desktop Notifications. In CHI Conference on Human Factors in Computing Systems (pp. 1-13).
- Abdou, A., Sood, E., Müller, P., & Bulling, A. (2022). Gaze-enhanced Crossmodal Embeddings for Emotion Recognition. Proceedings of the ACM on Human-Computer Interaction, 6(ETRA), 1-18.
- Müller, P., Dietz, M., Schiller, D., Thomas, D., Lindsay, H., Gebhard, P., … & Bulling, A. (2022, October). MultiMediate’22: Backchannel Detection and Agreement Estimation in Group Interactions. In Proceedings of the 30th ACM International Conference on Multimedia (pp. 7109-7114).
- Vozniak, I., Müller, P., Hell, L., Lipp, N., Abouelazm, A., & Müller, C. (2023). Context-empowered Visual Attention Prediction in Pedestrian Scenarios. In Proceedings of the IEEE/CVF Winter Conference on Applications of Computer Vision (pp. 950-960).
- Sharma, M., Chen, S., Müller, P., Rekrut, M., & Krüger, A. (2023). Implicit Search Intent Recognition using EEG and Eye Tracking: Novel Dataset and Cross-User Prediction. In Proc. International Conference on Multimodal Interaction (pp. 345-354).
- Withanage Don, D. S., Müller, P., Nunnari, F., André, E., & Gebhard, P. (2023). ReNeLiB: Real-time Neural Listening Behavior Generation for Socially Interactive Agents. In International Conference on Multimodal Interaction (pp. 507-516).